Explanatory Data Analysis group
Sander van Rijn
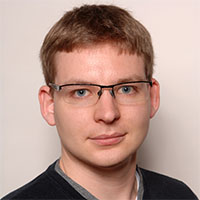
PhD cand.
Sander is currently developing surrogate-assisted optimization methods for the Data Mining High Volume Simulation Output (DAMIOSO) project. He seeks to combine traditional surrogates models with multi-fidelity fitness functions. In time, these methods are expanded to also make use of features which are extracted from the otherwise unused high volume output of computationally expensive CFD simulations.
Sander van Rijn, born in 1991 in Leiden, the Netherlands, received his Master degree of Computer Science at Leiden University, the Netherlands in 2016. He is currently pursuing a Ph.D. degree at the Leiden Institute of Advanced Computer Science (LIACS), Leiden University, where he is supervised by prof. dr. Thomas Bäck and dr. Matthijs van Leeuwen. His research project is a cooperation with the Honda Research Institute in Offenbach, Germany. His research interests are in developing evolutionary algorithms, Evolution Strategies in particular, that work with both surrogate models and computationally expensive fitness functions in multiple fidelities. He is also interested in the automated selection and tuning of modular evolutionary algorithms.
Selected recent publications
2022 |
|
Finding Efficient Trade-offs in Multi-Fidelity Response Surface Modeling. Engineering Optimization |
|
2020 |
|
MF2: A Collection of Multi-Fidelity Benchmark Functions in Python. Journal of Open Source Software vol.5(52), 2020. |
|
2018 |
|
Towards an Adaptive CMA-ES Configurator. In: Proceedings of the International Conference on Parallel Problem Solving from Nature (PPSN'18), 2018. |
|
Towards a Theory-Guided Benchmarking Suite for Discrete Black-Box Optimization: Profiling (1+λ) EA Variants on OneMax and LeadingOnes. In: Proceedings of the Genetic and Evolutionary Computation Conference (GECCO'18), pp 951-958, ACM, 2018. |
|
Multi-Fidelity Surrogate Model Approach to Optimization. In: Proceedings of the Genetic and Evolutionary Computation Conference (GECCO'18), ACM, 2018. |