Explanatory Data Analysis group
Dr. Matthijs van Leeuwen
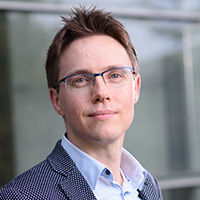
Associate professor & group leader
Associate professor & group leader
The short (compressed) version
Matthijs likes data, patterns, algorithms, and information theory. He strives for data mining and machine learning methods and results that are principled, interpretable, and exploit existing knowledge.
The longer version
Matthijs is associate professor and group leader at the Leiden Institute of Advanced Computer Science (LIACS), Leiden University. He is Programme Manager of the Master Computer Science, and affiliated with SAILS and DSRP, the university-wide research programmes for artificial intelligence (AI) and data science. His primary research interests are exploratory data mining and interpretable machine learning: how can we enable domain experts to explore and analyse their data, to uncover patterns and make predictions, and—ultimately—discover novel knowledge?
For this it is important that methods and results are explainable to domain experts, who may not be data scientists. His signature approach is to define and identify patterns that matter, i.e., succinct descriptions that characterise relevant structure present in the data. Which patterns matter strongly depends on the data and task at hand, hence defining the problem is one of the key challenges in his research. Information theoretic concepts such as the Minimum Description Length (MDL) principle have proven very useful to this end. Matthijs is also interested in interactive data mining, i.e., involving humans in the loop. Finally, he is interested in fundamental data mining research for real-world applications, both in science (e.g., life sciences, social sciences) and industry (e.g., manufacturing and engineering, aviation), as this is the best way to show that the theory works in practice.
Bio
Matthijs was previously a (tenure track) assistant professor (2017-2020) and senior researcher (2015-2017) at Leiden University, and a postdoctoral researcher at KU Leuven (2011-2015) and Universiteit Utrecht (2009-2011). He defended his Ph.D. thesis, titled Patterns that Matter, in February 2010, at Universiteit Utrecht. He won several best paper and reviewer awards at international conferences and was awarded NWO Rubicon, FWO Postdoc, NWO TOP2, and NWO TTW Perspectief grants. He is General Chair of the IDA Council and editorial board member of Data Mining and Knowledge Discovery. Further, he co-organised a number of international conferences and workshops, and co-lectured tutorials on 'Information Theoretic Methods in Data Mining'.
More information, including CV, at www.patternsthatmatter.org
Selected recent publications
In press |
|
Cross-Domain Graph Level Anomaly Detection. Transactions on Knowledge and Data Engineering, ACM |
|
2024 |
|
Conditional Density Estimation with Histogram Trees. In: Proceedings of the Conference on Neural Information Processing Systems (NeurIPS 2024), 2024. |
|
A Survey on Explainable Anomaly Detection. Transactions on Knowledge Discovery from Data vol.18(1), ACM, 2024. |
|
Human-guided Rule Learning for ICU Readmission Risk Analysis. In: Proceedings of the Workshop on AI and Data Science for Healthcare (AIDSH) at KDD 2024, 2024. |
|
Graph Neural Networks based Log Anomaly Detection and Explanation. In: Proceedings of the 2024 IEEE/ACM 46th International Conference on Software Engineering: Companion Proceedings, pp 306-307, ACM, 2024. |
|
2023 |
|
Evaluating Cluster-Based Synthetic Data Generation for Blood-Transfusion Analysis. Journal of Cybersecurity and Privacy vol.3(4), pp 882-894, MDPI, 2023. |
|
WEARDA: recording wearable sensor data for human activity monitoring. Journal of Open Research Software vol.11(1), 2023. |
|
The added value of ferritin levels and genetic markers for the prediction of haemoglobin deferral. Vox Sanguinis vol.118(10), pp 825-834, 2023. |
|
Explainable Contextual Anomaly Detection using Quantile Regression Forests. Data Mining and Knowledge Discovery, Springer |
|
Novel approach for phenotyping based on diverse top-k subgroup lists. In: Proceedings of the Conference on Artificial Intelligence In Medicine (AIME 2023), Springer, 2023. |
|
Discovering Diverse Top-k Characteristic Lists. In: Proceedings of the 21st International Symposium on Intelligent Data Analysis (IDA 2023), Springer, 2023. |
|
Discovering Rule Lists with Preferred Variables. In: Proceedings of the 21st International Symposium on Intelligent Data Analysis (IDA 2023), Springer, 2023. |
|
Defining migraine days, based on longitudinal E-diary data. Cephalalgia |
|
Unsupervised Discretization by Two-dimensional MDL-based Histogram. Machine Learning, Springer |
|
Generating synthetic mixed discrete-continuous health records with mixed sum-product networks. Journal of the American Medical Informatics Association vol.30(1), Oxford University Press, 2023. |